How Professionals Identify the Best New Customer with Predictive Models
Written on .
Marketing has always been about trying to understand what the customer wants. With recent advancements in big data, data analytics, and machine learning, predicting customer behavior has become achievable. This technological expansion has given marketers more powerful tools to predict customer preferences and buying patterns.
6 minute read
Table of Contents
- How Professionals Identify the Best New Customer with Predictive Models

If marketers could accurately make customer behavior predictions, it would make life easier for everyone. Machine learning and propensity models are two tools that brands can use to anticipate and optimize the impact of their marketing tactics. Recently, propensity modeling has become a popular tool for predicting an individual's likelihood of taking a specific action, like purchasing within a certain timeframe.
Grand View Research reports that the predictive analytics market will continue to grow at a CAGR of 23.2% through 2025 as more companies leverage predictive modeling for better decision-making in their marketing endeavors.
So, what is predictive modeling? Predictive modeling is a method of using statistical techniques to make predictions about future events. This type of modeling is often used to drive customer predictions in marketing. It is designed around how to predict customer behavior. For example, predictive modeling can make customer purchase behavior predictions from payment datasets to determine which customers will most likely purchase a product within the next month.
Predictive analytics customer behavior modeling is based on the idea that past behavior is a good indicator of future behavior. This means that if we can identify the factors influencing a customer's purchase decision, we can use this information to predict future behavior.
Information on your ideal customers – for example, their buying habits, attitudes, and demographics – is necessary to create and carry out marketing programs that will work well. This data can help develop targeted promotional campaigns, as well as other programs aimed at increasing customer retention, loyalty, or boosting sales. This knowledge, made possible by propensity modeling, comes in handy when creating a prediction model of a new customer's behavior profile.
What is the Best 'New' Customer?
The best new customer for your business is an individual whose behavior profile closely matches your existing customers who have registered a high lifetime value (LTV). You'll need to build a propensity model to find your best new customers. Customer behavior prediction models forecast the likelihood that someone will perform a specific action--such as buying something, signing up for a newsletter, or leaving (churning)--based on their past behavior.
By analyzing patterns in data, you can understand how likely someone will take the desired action. You can then calculate a propensity score - a probabilistic estimation of the likelihood that a customer will perform those actions. Using this score, you can grade that customer's value and use this data to predict who among your potential leads could become your newest best customer.
Steps to Find Your Best 'New' Customer with Propensity Models
So, how can you leverage propensity score models to find your best ‘new’ customer? Here are four steps you can follow:
Analyze your current customer base
Take a close look at your existing customer base to figure out:
- Who they are - the age and gender of individual consumers or industry and business size for corporate customers.
- What they think and believe - their interests, opinions of you and your product.
- How they behave - their historical data, including which products they buy, where, when, and how they pay.
With this information, you can create a behavior profile for your ideal new customer.
Define what makes a customer valuable
Before anything, you must figure out what characteristics make a customer valuable to your company. For example, is it someone who buys high-margin items or only pays the sticker price? Is it better to have customers that order fewer oversized items or multiple small ones? Is a customer's value measured by whether or not they cancel or amend orders?
Does your business prefer customers who purchase and pay on time without needing reminders or extensive after-sales service? Identify the attributes most important to your brand and give each attribute different levels of importance.
Choose the criteria for building the propensity model of customers that will bring you revenue
To find these individuals, use predictive modeling to score each customer according to how likely they are to exhibit the desired behavior. The model will analyze all available data on your customers and potential customers to generate a propensity score.
Create the propensity model for the best 'new' customer
This will help you identify new prospects more likely to behave like your best customers. You can use the model to score new leads and prioritize those most likely to turn into high-value customers.
Integrate Into Operational Processes and Update or Create Versions
Once the propensity model is created for a particular outcome the outputs need to be integrated into the operational data flows so they can be acted upon. This will help you achieve scale with the insights. Then create different versions for other outcomes or segments and look to update the model based on the needs of the day for greater returns and more scale.
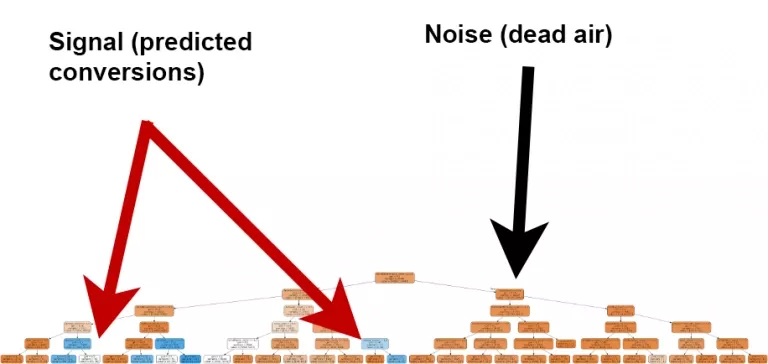
Using Propensity Modeling for the Best 'New' Customer Profile
Once you have identified your best "new" customer profile, you can use propensity modeling to score new leads and prioritize those most likely to turn into high-value customers.
Good propensity score models must be:
- Dynamic - Models should always consider the latest data to advance with trends, adapt, and learn.
- Scalable - Models shouldn't just be designed for one use only; they should be capable of producing a range of predictions.
- Adaptive - Data pipelines need to regularly ingest new data, validate it, and deploy changes so that models stay up-to-date.
You can deploy one or more of these propensity score model types:
- Model for propensity to convert/purchase
- Model for propensity to engage
- Model for propensity to churn
- Model for customer lifetime value prediction using machine learning
The propensity to convert/purchase model lets you know which customers will be more inclined or less likely to buy your services or products or carry out some target action like subscribing to a newsletter. After figuring out which of your customers are willing to purchase from you, you can send them customized offers.
The propensity to engage model lets you analyze the likelihood of your leads and customers taking proactive action. For example, it could be a score that displays which website visitors are most likely to click on an ad.
The propensity to churn model allows you to discern which potential and current customers may leave your company. By measuring this estimation, you can then decide whether or not a re-engagement campaign would be beneficial to keep them as a customer.
How to predict customer lifetime value: Lifetime Value: Total Gross Revenue - Total Cost
Predicting customer lifetime value (CLV) using the customer lifetime value model estimates the total amount of money a customer will spend on your business during their lifetime engagement with your brand. You can use this information to see which marketing campaigns produce customers with the highest CLV so you know where it's worth investing more in the future.
You can merge distinct propensity models in certain situations to make more intelligent campaign choices. For example, you could offer a bigger discount to customers who are likely to churn but have a higher lifetime value.
Use Case: Find A High-LTV New Customer with Predictive Modeling
Acquiring customers involves a lot of expenses (like advertising, promotions, discounts, etc.) But sometimes, some customers cost more money than they're worth in terms of lifetime value. You need to be able to identify these behavior patterns and segment your customers so that you can take appropriate action. You can do this by building machine learning predictive models that will predict your customers' lifetime value.
These steps should help you build an effective predictive model:
- Define an appropriate time frame for Customer Lifetime Value calculation (e.g., number of months, years)
- Identify the parameters you are going to use to predict the new customers with the highest LTV (e.g., avg. number of purchases, avg. order value, the frequency of purchases)
- Train the predictive model and calculate LTV
- Finalize and run the predictive model. Apply clustering and have three segments (low LTV, mid LTV, or high LTV). The number of segments will depend on your business dynamics and goals.
- Check the successful results for the high LTV segment. Confirm that this predictive model works for your business.
With this predictive model in hand, you'll be able to segment the leads by the probability to make recurring purchases, and prioritize those most likely to turn into high-value customers.
Conclusion
By understanding how to apply predictive modeling, you can more intelligently segment your customers, personalize campaigns, and allocate resources for maximum ROI. Predictive analytics models are a must-have for a successful decision-making process. They help to reduce risks and costs and define the audience, message, and expected outcome before execution.
Predictive modeling can be applied to many key parameters that impact the final outcome for your business (e.g., purchase, average order value, acquisition cost, LTV). The models are beneficial in defining which audience segment will bring the highest outcome to the business and engage them to close deals.

Elsa Petterson
Partner success manager @ Put It Forward