Get Control by Automating Data Quality Processes at Scale
Accessible intelligent solutions that let you be in control of your data quality across the organization.
- Configure AI powered data quality detection to scale
- Built in anomaly and error detection to reveal issues
- Identify hidden issues and events within the data

Requirements for Successful Data Quality Automation
Identify Hidden Patterns

Easy Model Configuration
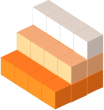
What If Data Quality Scenarios
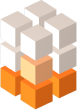
Explainable Decisions Guide
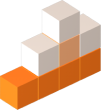
Your Powerful AI Data Quality Workbench
Designed to be used by the professional that needs to include test multiple different scenarios quickly and at scale.
-
Quickly adjust the models with new parameters to identify hidden patterns and correlations
-
Predict impacts of correlations within the data and feed into operational workflows to trigger business events


Capture and See What's Going On In The Data Flows
Work directly with predictive lead scoring analytics models or the data scientists on your team to define which criteria the machine learning should consider.
-
Quickly know the quality of your input data before it proliferates across your enterprise
-
Assign relative quality scores to records in flight between systems
-
Drill down to the field level as it's flowing through
Learn More About How To Succeed With Put It Forward Delphi
5 Keys to Successful Data Governance
Once your data is created and stored you need to plan for the management of its lifecycle. A data warehouse like Snowflake requires a solid governance plan.
What is Data Integration?
Data integration comes in many styles and formats that can easily be confusing to the novice. Learn about the different types and when to use each for your benefit.
Scaling by AI Automation
Repeatable patterns for success to scale by automation and AI driven processes. Learn from how we’ve managed to leverage these two concepts for scale.
Ready to Take the Next Step With Predictive Lead Scoring?
Control Your Data Story - Why Partner With Put It Forward?
Proven Scale
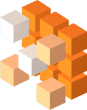
Independent Roadmap
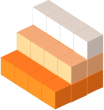
Complete Data Platform
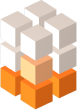
Secure and Compliance Ready

Put It Forward - An Integrated Solution Suite
Modern tools that run alone or together to help you scale the intelligent data story

Platform Manager
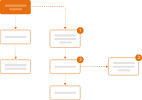
Integration Designer

Delphi - Predictive
Key Features Of A Predictive Data Analytics Platform

Dynamic Prediction Modelling

No Code Configuration

Certified Connectors

Embedded 360 Analytics

Automated Quality Analysis

Easy Transformations

In-flight Governance

Secure On-Premise Connection
Frequently Asked Questions about Data Quality
Data quality automation is the process of using machine learning and artificial intelligence to ensure that data is of high quality and free of potential errors.
The process can include steps like making sure that data is complete, accurate, and free of duplicates. Intelligent data quality automation helps organizations improve the quality of obtained data by catching and fixing errors before they become a problem.
An automated data quality platform by Put It Forward gives organizations full control over their data and its quality.
With its use, organizations can test multiple different scenarios efficiently and identify hidden patterns or correlations by adjusting the data models with new parameters.
The automated data quality platform can also be used to predict the impacts of correlations and modify operational workflows to trigger specific business events.
There are many data quality automation benefits, including the ability to:
- Automate data quality checks and improve their accuracy and efficiency,
- Streamline data management processes,
- Improve data governance and control,
- Reduce costs associated with manual data quality management processes,
- Increase consistency and accuracy of data across systems and departments,
- Reduce the need for manual data quality checks,
- Improve data quality by identifying and correcting errors before data is entered into systems.